My Story
As a dedicated student, I graduated in June 2018 with a BS Degree in Electrical and Electronics Engineering with honors. I developed hobbies, such as swimming, reading, and hiking, since I felt there is more out there for personal growth. I think you see life through a different lens when you enrich your perspective by extending the exposure to a spectrum from psychology and philosophy to mathematics and engineering. In my appreciation of diverse interests, I have never stopped exploring possibilities in human and computer learning and signal processing.
​
In the end, considering my interest in the human brain, I am currently focusing mainly on applications based on neural networks during my Master's Degree program at Bilkent University Department of Computer Science. After graduating with an MSc. Degree, Having discovered my interest in the topic and desire for searching more, I would like to be an active part of the science world. I will be still searching for more and I would like it to be highly related to how we can use learning machines to go further and further.
Research Areas
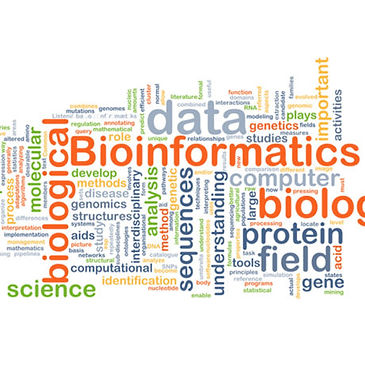
Computational Genomics and Gene Risk Assestment
Current methods developed for risk gene identification is one-way, meaning that only risk genes for a single disorder are proposed. A common way of finding risk genes for comorbid disorders, such as ASD and ID, is to run a model twice for each disorder and then intersect the results, which fails to capture the common signal between two disorders. Using such a technique fails to utilize the genetic circuitry shared by these disorders.
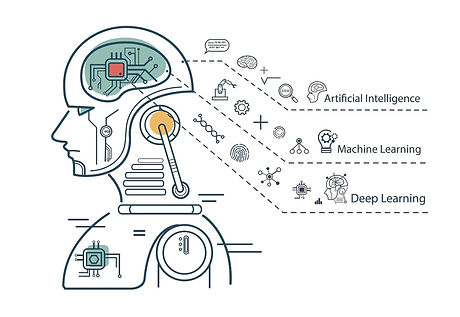
Data mining & Machine Learning
Deep learning has become one of the most successful and popular machine learning algorithms in recent years since deep architectures can capture nonlinear patterns and process big data more effectively. With developments in hardware technology, deep networks quickly replace the state-of-the-art performance of many machine learning tasks in numerous disciplines including biology and genomics. Although they are less interpretable than other machine learning methods, their ability to capture nonlinear patterns in the data makes them preferable for most of the data desired to be analyzed.
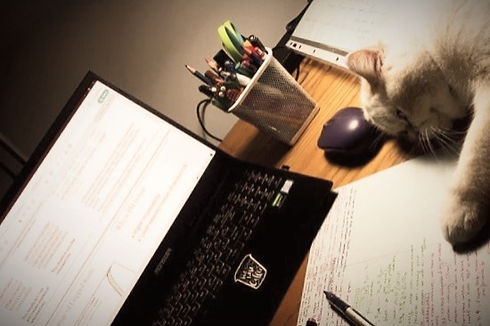